Learn
Dive into resources from the commute experts to learn about the science behind Pave Commute, check out our case studies, and discover how your organization can improve the commuter experience.
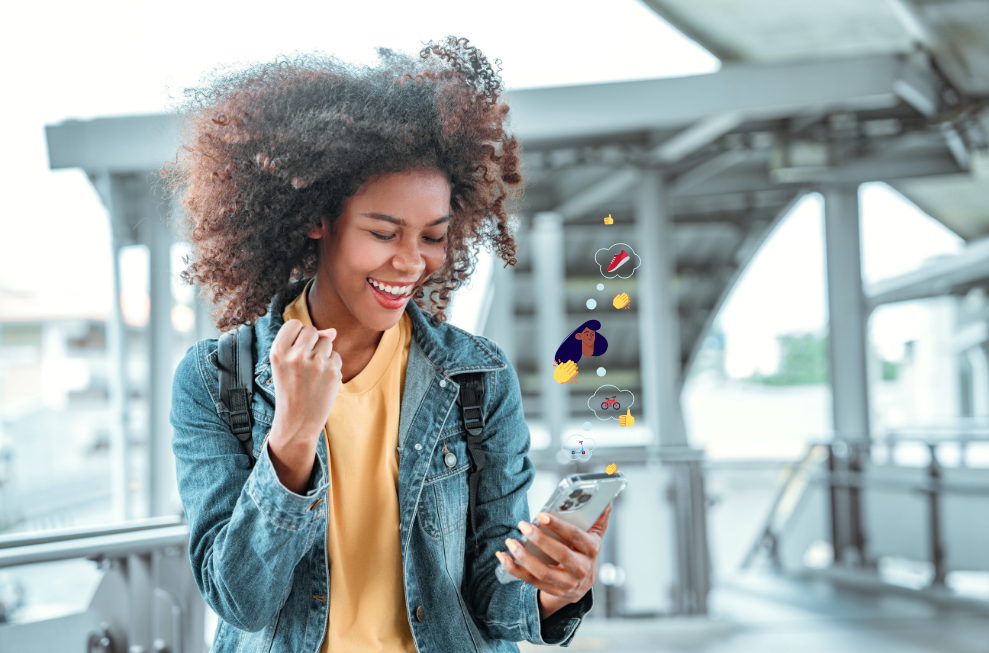
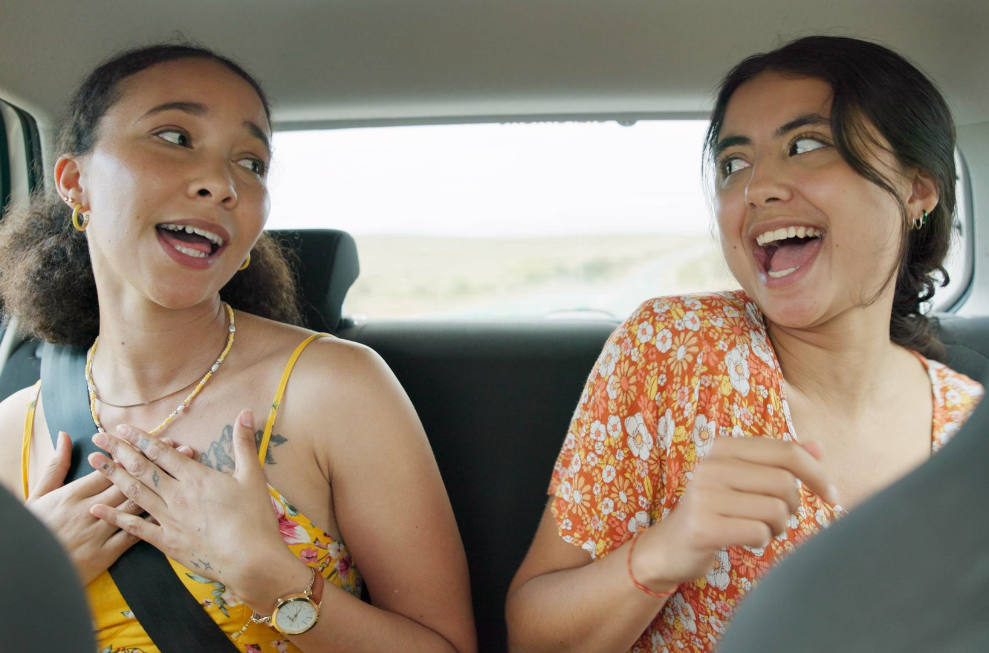
How to Find a Free Carpool to Work
May 8, 2025
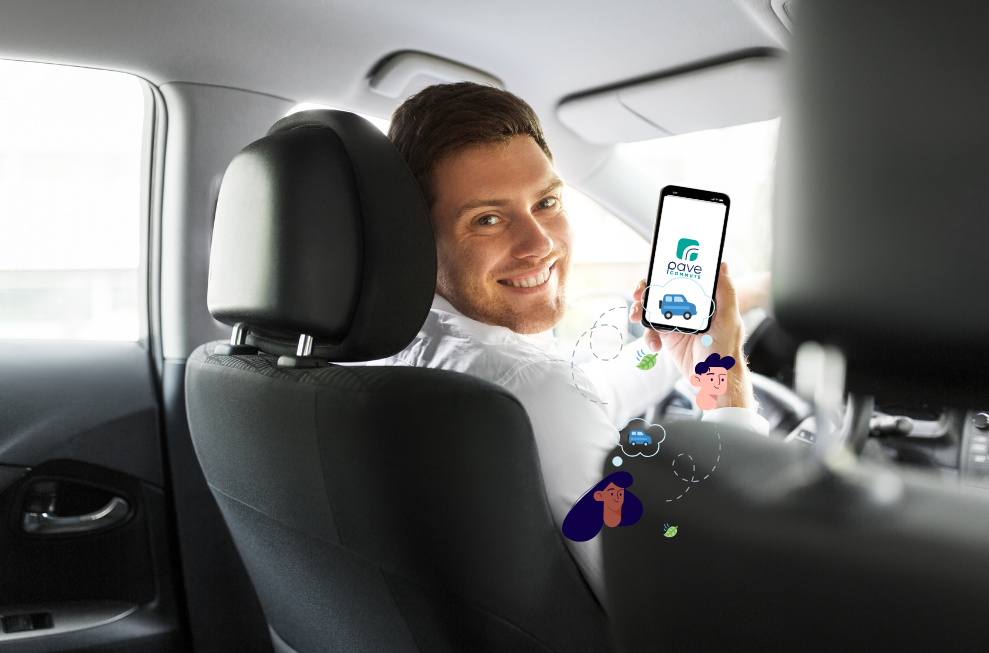
The Best Carpool App for Your Commute
April 22, 2025
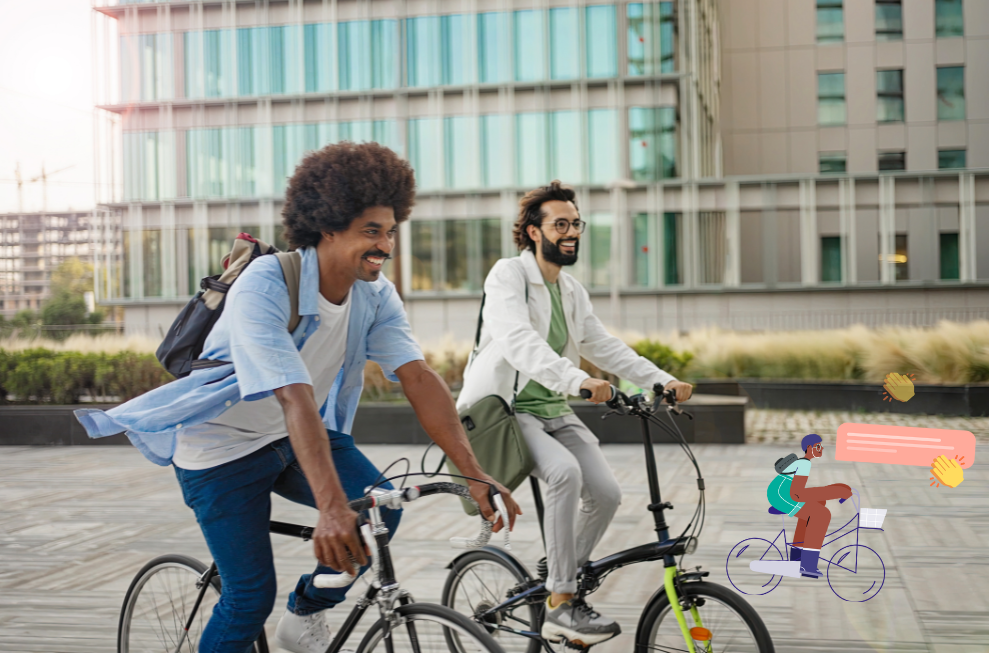
Making Friends at Work – Starting on Your Commute
April 15, 2025
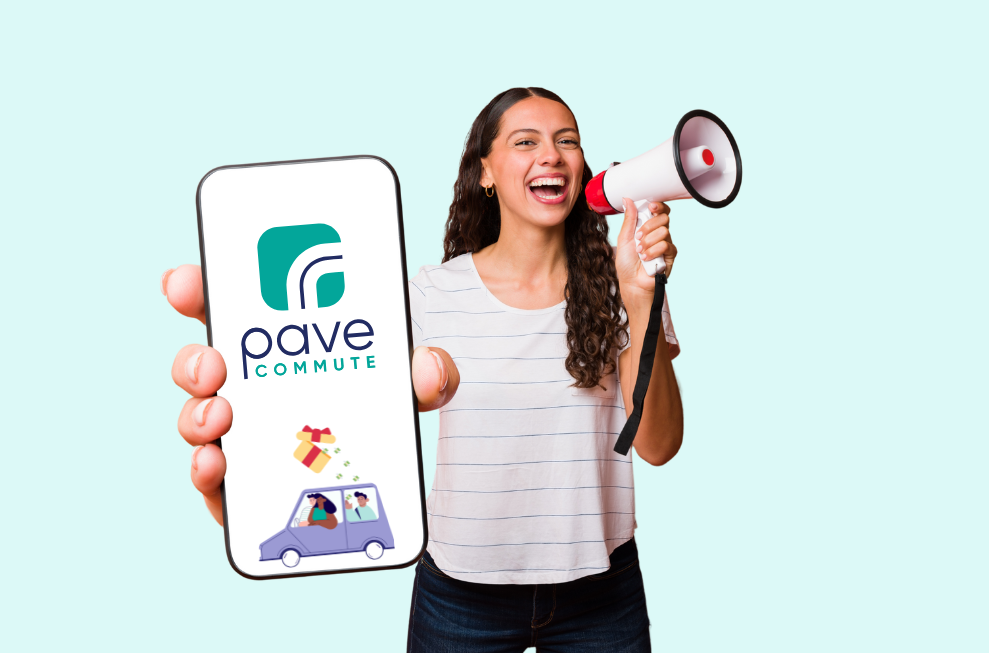
The Best Carpooling App for Work
April 7, 2025
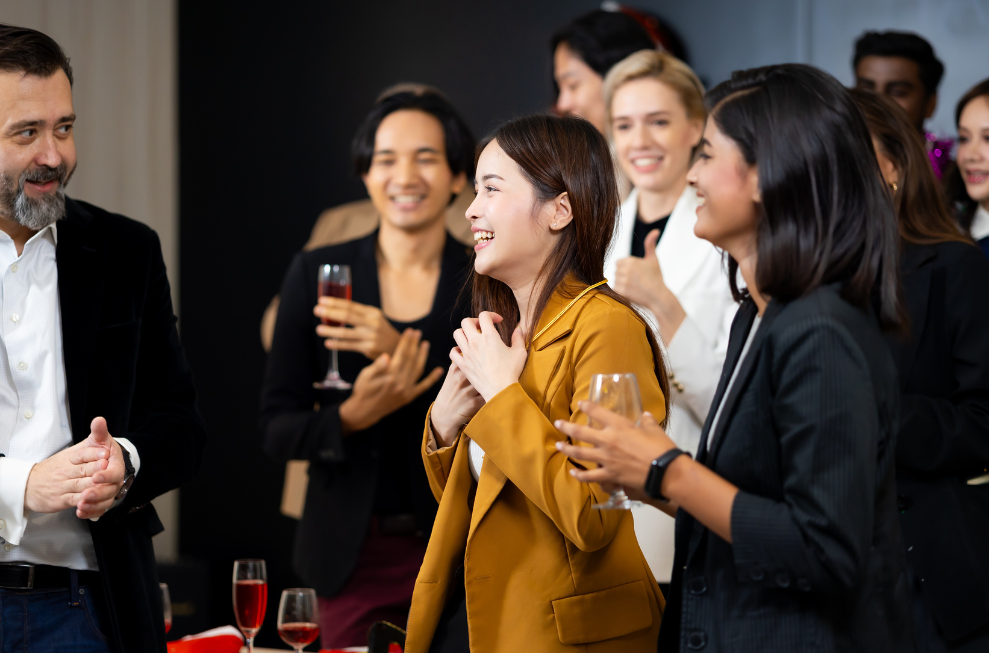